DeepHot is a term that combines the fascinating world of deep learning with the hottest trends in artificial intelligence (AI). As technology continues to evolve, the importance of understanding deep learning becomes increasingly critical for both individuals and businesses. In this comprehensive guide, we will explore the key concepts, applications, and future of deep learning, providing you with the knowledge you need to stay ahead in this rapidly changing landscape.
Deep learning, a subset of machine learning, utilizes neural networks to analyze vast amounts of data and make predictions or decisions without human intervention. It has revolutionized various fields, including healthcare, finance, and entertainment, by enabling machines to learn and adapt in ways that were previously unimaginable. This article will unravel the complexities of deep learning and highlight its significance in today’s AI-driven world.
In addition to understanding deep learning itself, it is essential to stay updated on the latest hot topics in the field. From advancements in natural language processing to breakthroughs in computer vision, the AI landscape is constantly evolving. This guide aims to provide insights into these developments, helping you navigate the intricate world of AI and deep learning.
Table of Contents
- What is Deep Learning?
- History of Deep Learning
- How Does Deep Learning Work?
- Applications of Deep Learning
- Hot Topics in AI
- Challenges in Deep Learning
- Future of Deep Learning
- Conclusion
What is Deep Learning?
Deep learning is a branch of artificial intelligence that mimics the way humans learn. It uses neural networks with many layers (hence "deep") to analyze data and make decisions. Deep learning has been particularly effective in tasks such as image recognition, speech recognition, and natural language processing.
Key Features of Deep Learning
- Ability to learn from large amounts of data.
- Use of neural networks to model complex patterns.
- Minimal human intervention required for training.
- High accuracy in predictions and classifications.
History of Deep Learning
The roots of deep learning can be traced back to the 1940s, with the development of the first neural networks. However, significant advancements have occurred in the last decade, driven by increased computational power and the availability of large datasets.
Some key milestones in the history of deep learning include:
- 1943: McCulloch and Pitts create the first artificial neuron.
- 1986: Backpropagation algorithm introduced by Rumelhart, Hinton, and Williams.
- 2012: AlexNet wins the ImageNet competition, showcasing the power of deep learning.
- 2016: Google's AlphaGo defeats the world champion in the game of Go.
How Does Deep Learning Work?
Deep learning models are built using artificial neural networks that consist of interconnected nodes (neurons). These networks are structured in layers, with input layers receiving data, hidden layers processing it, and output layers delivering results.
The training process involves feeding the model large datasets, allowing it to adjust the weights of the connections between neurons to minimize prediction errors. This iterative process is known as training the model.
Types of Neural Networks
- Convolutional Neural Networks (CNNs): Ideal for image processing.
- Recurrent Neural Networks (RNNs): Suitable for sequential data, such as time series.
- Generative Adversarial Networks (GANs): Used for generating new data similar to existing datasets.
Applications of Deep Learning
Deep learning has a wide array of applications across various domains:
- Healthcare: Assisting in diagnostics and personalized treatment.
- Finance: Fraud detection and algorithmic trading.
- Entertainment: Content recommendation systems in streaming services.
- Autonomous Vehicles: Object detection and navigation systems.
Hot Topics in AI
Staying updated on hot topics in AI is crucial for professionals and enthusiasts alike. Some of the current hot topics include:
- Natural Language Processing (NLP): Enhancements in understanding and generating human language.
- Explainable AI (XAI): Making AI systems more transparent and interpretable.
- AI Ethics: Addressing biases and ensuring fairness in AI systems.
- Federated Learning: Collaborative learning without sharing raw data.
Challenges in Deep Learning
Despite its remarkable capabilities, deep learning faces several challenges:
- Data Privacy: Ensuring user data is protected during training.
- Computational Costs: High resource requirements for training complex models.
- Overfitting: Models performing well on training data but poorly on unseen data.
- Interpretability: Difficulty in understanding how models make decisions.
Future of Deep Learning
The future of deep learning appears promising, with continuous advancements expected. Some trends to watch include:
- Integration of deep learning with other AI techniques.
- Increased focus on ethical AI and responsible usage.
- Growing demand for skilled professionals in the field.
- Development of more efficient algorithms to reduce resource consumption.
Conclusion
In conclusion, deep learning is a vital component of the AI landscape, driving innovations across various sectors. Understanding its principles and applications is essential for anyone looking to navigate the complexities of technology today. We encourage you to share your thoughts in the comments below and explore more articles on our site to deepen your knowledge of AI and deep learning.
Thank you for reading! We look forward to welcoming you back for more insightful content.
You Might Also Like
MMS Subhashree Sahu: The Rising Star In The SpotlightMother Of Elvis Presley: The Life And Legacy Of Gladys Presley
Movierulztoday: The Ultimate Guide To Movie Streaming
Jimena Gomez: A Rising Star In The Entertainment Industry
Prichard Colon Married: A Deep Dive Into His Life And Relationships
Article Recommendations


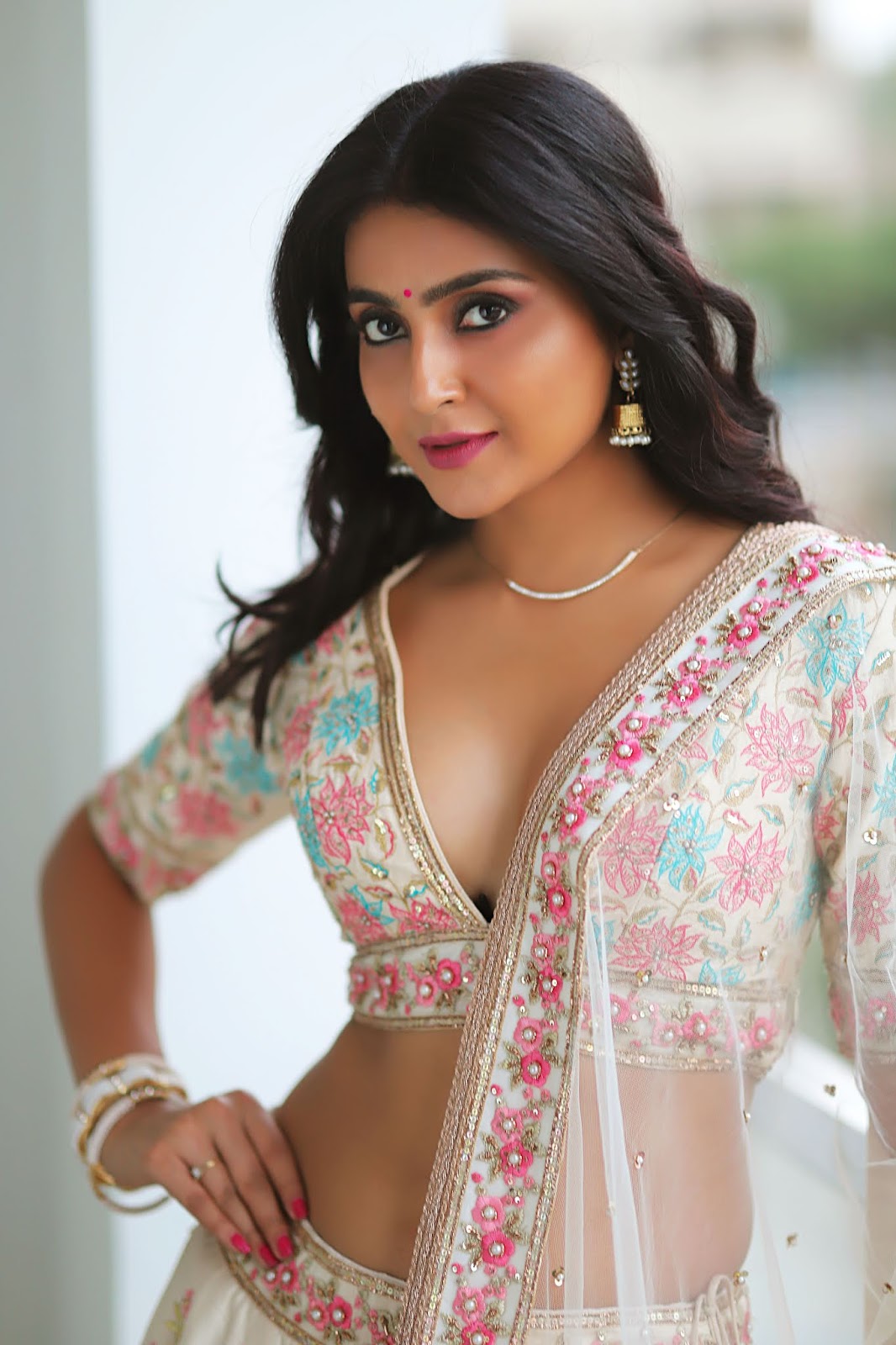